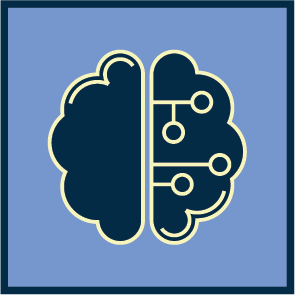
AI-based methodology & Project structure
To unlock valuable plant metabolites for society, deCYPher needs to address five key challenges:
- The use of digital tools for biotechnology
- Suitable infrastructure for managing bio-based innovation data
- An integrated approach in bioprocess development
- Efficient identification and expression of CYPs in microbes
- Addressing stakeholders’ needs
Solving these issues requires a cutting-edge, integrated, and combinatorial approach that seeks to innovate how bioprocesses are developed. deCYPher will develop a standardised artificial intelligence (AI) and machine learning (ML) platform for biotech applications as well as guiding bioprocesses across all steps of the development chain.
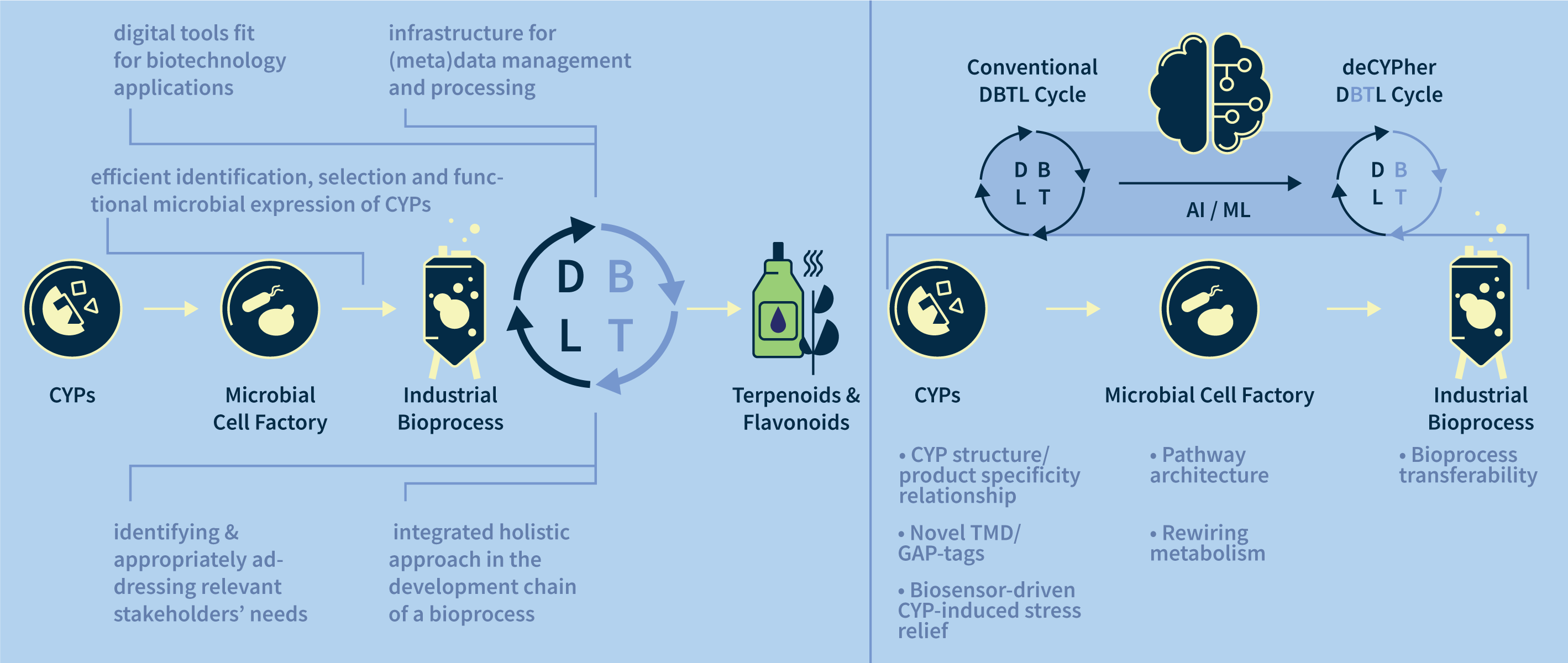
On the left, five main challenges are identified to enable sustainable bio-based production of terpenoids and flavonoids. On the right, artificial intelligence (AI) and machine learning (ML) techniques are fully integrated into the Design-Build-Test-Learn (DBTL) cycles throughout the bioprocess development chain. CYP refers to cytochrome P450 enzymes.
Project structure and work packages (WPs):
The workplan is bookended with three work packages addressing tasks outside of the research work: administration/management (WP 7); communication/dissemination/exploitation, including open-science and citizen engagement (WP 6); and data management (WP5).
The research and innovation content of deCYPher is covered by work packages 1-4 with dedicated partners in the consortium acting as lead for specific work packages and tasks. Thematically these are structured around:
- Learning techniques fit for industrial biotechnology (WP1)
- Bioprospecting and functional expression of CYPs (WP2)
- Microbial cell factories for oxygenated flavonoids and terpenoids (WP3)
- Sustainable bio-based production of flavonoids and terpenoids (WP4)
The chart below shows the unidirectional and bi-directional relationships between the different WPs.
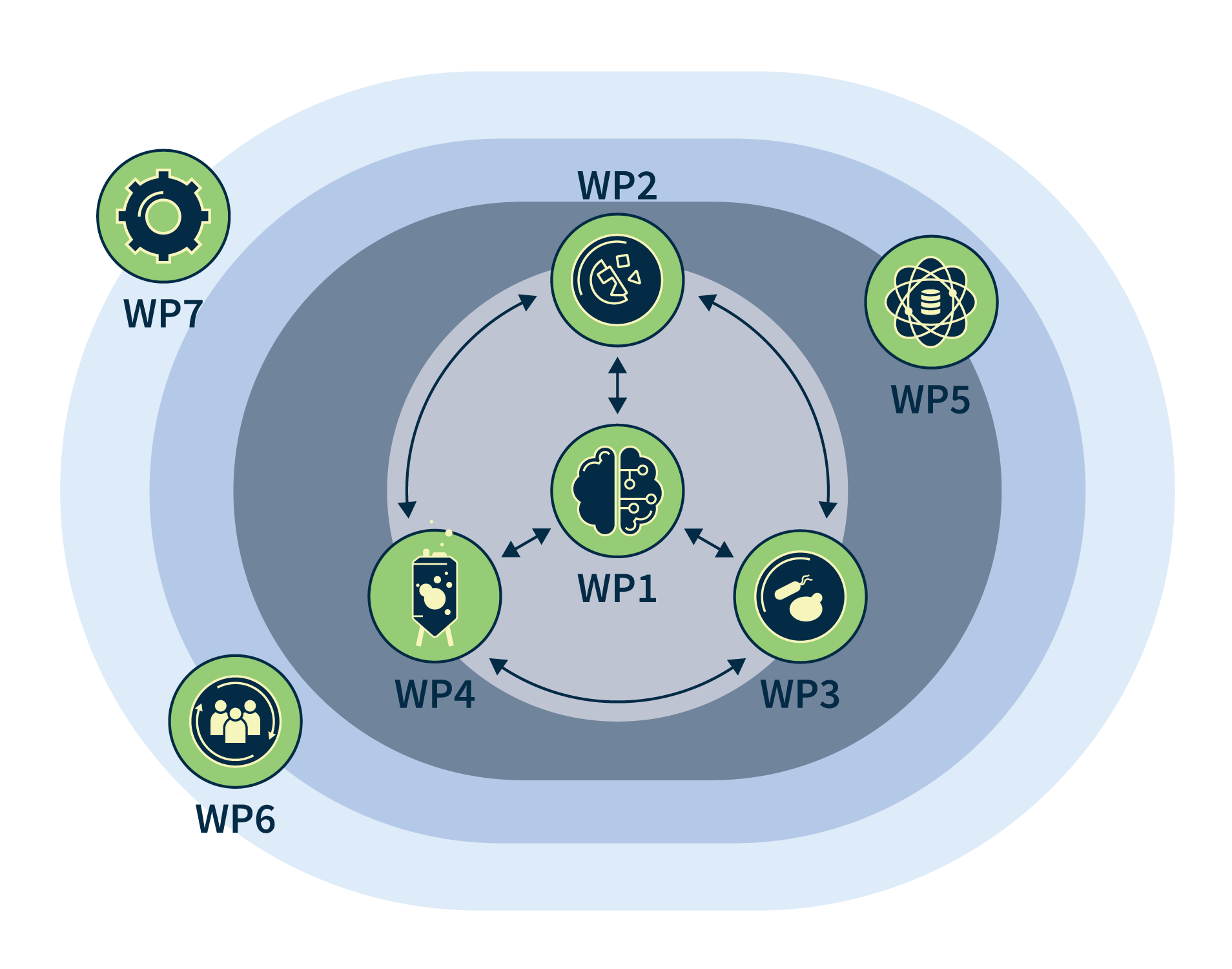